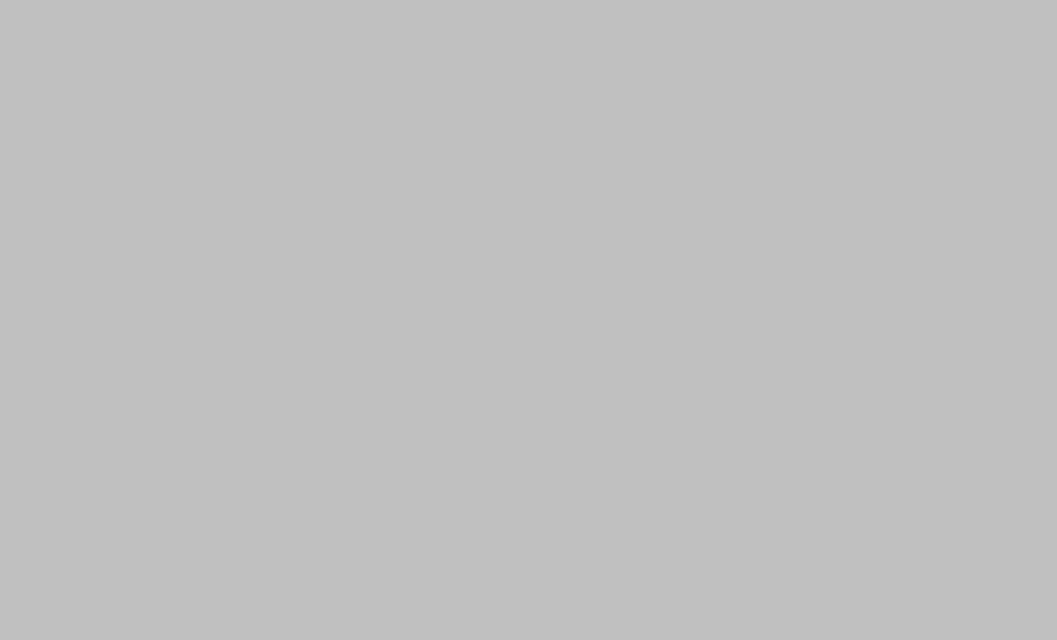
zeit.de
Teacher Exodus Looms in North Rhine-Westphalia Amidst Excessive Workloads
A survey of 3,105 teachers in North Rhine-Westphalia found that 38% are considering leaving their jobs due to excessive workloads, with over 40% working 41-50 hours weekly and 37% working over 50 hours, while the government cites 12,000 new hires in the last 36 months as a countermeasure.
- What are the root causes of the excessive workload reported by teachers in the survey?
- The survey highlights a critical staffing shortage in North Rhine-Westphalia's schools. The high percentage of teachers considering resignation, coupled with the excessive workload reported, suggests systemic issues impacting teacher retention. While 12,000 new hires, including over 10,000 teachers, have been made in the past 36 months, this may be insufficient to counter the high attrition rate.
- What are the immediate consequences of the high percentage of teachers in North Rhine-Westphalia considering leaving their jobs?
- A recent survey of 3,105 teachers in North Rhine-Westphalia revealed that 38% are considering leaving their jobs, with an additional 33% occasionally contemplating it. The main reason cited is excessive working hours; less than 10% work within their contracted hours, while over 40% work 41-50 hours weekly, and 37% work over 50 hours.", A2="The survey highlights a critical staffing shortage in North Rhine-Westphalia's schools. The high percentage of teachers considering resignation, coupled with the excessive workload reported, suggests systemic issues impacting teacher retention. While 12,000 new hires, including over 10,000 teachers, have been made in the past 36 months, this may be insufficient to counter the high attrition rate.", A3="The long-term consequences of this teacher shortage could include larger class sizes, reduced educational quality, and potential burnout among remaining staff. The government's response focuses on ongoing efforts to alleviate teacher workload, but the survey results indicate these measures may be insufficient. Addressing systemic issues, like workload distribution and teacher support, is crucial for long-term teacher retention.", Q1="What are the immediate consequences of the high percentage of teachers in North Rhine-Westphalia considering leaving their jobs?", Q2="What are the root causes of the excessive workload reported by teachers in the survey?", Q3="What long-term strategies are needed to address the teacher shortage and improve working conditions in North Rhine-Westphalia's schools?", ShortDescription="A survey of 3,105 teachers in North Rhine-Westphalia found that 38% are considering leaving their jobs due to excessive workloads, with over 40% working 41-50 hours weekly and 37% working over 50 hours, while the government cites 12,000 new hires in the last 36 months as a countermeasure.", ShortTitle="Teacher Exodus Looms in North Rhine-Westphalia Amidst Excessive Workloads")) ######### print(df.head()) ######### print(df.describe()) ######### print(df.info()) ######### print(df.columns) ######### print(df.shape) ######### print(df.dtypes) ######### print(df.isnull().sum()) ######### print(df.nunique()) ######### print(df.tail()) ######### print(df.sample(5)) ######### print(df.sort_values('column_name')) ######### print(df.groupby('column_name').mean()) ######### print(df.corr()) ######### print(df.plot()) ######### print(df.plot.hist()) ######### print(df.plot.scatter('column_name1', 'column_name2')) ######### print(df.plot.box()) ######### print(df.plot.kde()) ######### print(df.plot.area()) ######### print(df.plot.bar()) ######### print(df.plot.pie('column_name')) ######### print(df.plot.hexbin('column_name1', 'column_name2')) ######### print(df.plot.imshow()) ######### print(df.plot.line()) ######### print(df.plot.step()) ######### print(df.plot.violin()) ######### print(df.plot.andrews_curves('column_name')) ######### print(df.plot.parallel_coordinates('column_name')) ######### print(df.plot.radviz('column_name')) ######### print(df.plot.scatter_matrix()) ######### print(df.style.highlight_max(axis=0)) ######### print(df.style.highlight_min(axis=1)) ######### print(df.style.background_gradient()) ######### print(df.style.bar()) ######### print(df.style.format('{:.2f}')) ######### print(df.to_json()) ######### print(df.to_csv()) ######### print(df.to_html()) ######### print(df.to_excel('output.xlsx')) ######### print(pd.read_csv('input.csv')) ######### print(pd.read_excel('input.xlsx')) ######### print(pd.read_json('input.json')) ######### print(pd.read_html('input.html')) ######### print(pd.read_clipboard()) ######### print(pd.to_datetime(df['column_name'])) ######### print(pd.to_numeric(df['column_name'])) ######### print(pd.to_timedelta(df['column_name'])) ######### print(pd.cut(df['column_name'], bins=5)) ######### print(pd.qcut(df['column_name'], q=4)) ######### print(pd.value_counts(df['column_name'])) ######### print(pd.concat([df1, df2])) ######### print(pd.merge(df1, df2, on='column_name')) ######### print(pd.pivot_table(df, values='column_name1', index='column_name2', columns='column_name3')) ######### print(pd.crosstab(df['column_name1'], df['column_name2'])) ######### print(pd.factorize(df['column_name'])) ######### print(pd.get_dummies(df['column_name'])) ######### print(pd.melt(df, id_vars=['column_name'])) ######### print(pd.wide_to_long(df, stubnames='column_name', i=['column_name1', 'column_name2'], j='column_name3')) ######### print(pd.explode(df['column_name'])) ######### print(pd.unique(df['column_name'])) ######### print(pd.isna(df['column_name'])) ######### print(pd.notna(df['column_name'])) ######### print(pd.fillna(df['column_name'], value=0)) ######### print(pd.dropna(df)) ######### print(pd.duplicated(df)) ######### print(pd.drop_duplicates(df)) ######### print(pd.replace(df['column_name'], to_replace='old_value', value='new_value')) ######### print(pd.map(df['column_name'], func)) ######### print(pd.apply(df, func, axis=0)) ######### print(pd.applymap(df, func)) ######### print(pd.select_dtypes(include=['number'])) ######### print(pd.select_dtypes(exclude=['number'])) ######### print(pd.set_option('display.max_rows', 100)) ######### print(pd.set_option('display.max_columns', 100)) ######### print(pd.reset_option('display.max_rows')) ######### print(pd.reset_option('display.max_columns')) ######### print(pd.describe_option('display.max_rows')) ######### print(pd.describe_option('display.max_columns')) ######### print(pd.test()) ######### print(pd.__version__) ######### print(pd.show_versions()) ######### print(pd.read_fwf('input.txt')) ######### print(pd.read_table('input.txt')) ######### print(pd.read_stata('input.dta')) ######### print(pd.read_sas('input.sas7bdat')) ######### print(pd.read_feather('input.feather')) ######### print(pd.read_parquet('input.parquet')) ######### print(pd.read_hdf('input.h5')) ######### print(pd.read_gbq('query', project_id='project_id')) ######### print(pd.to_datetime(df['column_name'], format='%Y-%m-%d')) ######### print(pd.to_datetime(df['column_name'], unit='s')) ######### print(pd.to_datetime(df['column_name'], errors='coerce')) ######### print(pd.to_timedelta(df['column_name'], unit='h')) ######### print(pd.to_timedelta(df['column_name'], errors='ignore')) ######### print(pd.cut(df['column_name'], bins=[0, 10, 20, 30], labels=['low', 'medium', 'high'])) ######### print(pd.qcut(df['column_name'], q=4, labels=['1st', '2nd', '3rd', '4th'])) ######### print(pd.value_counts(df['column_name'], normalize=True)) ######### print(pd.concat([df1, df2], axis=1)) ######### print(pd.merge(df1, df2, how='left', on='column_name')) ######### print(pd.pivot_table(df, values='column_name1', index='column_name2', columns='column_name3', aggfunc='sum')) ######### print(pd.crosstab(df['column_name1'], df['column_name2'], normalize='index')) ######### print(pd.factorize(df['column_name'], sort=True)) ######### print(pd.get_dummies(df['column_name'], prefix='column_name')) ######### print(pd.melt(df, id_vars=['column_name'], var_name='variable', value_name='value')) ######### print(pd.wide_to_long(df, stubnames=['column_name'], i=['column_name1', 'column_name2'], j='column_name3', sep='_', suffix='\d+')) ######### print(pd.explode(df['column_name'], ignore_index=True)) ######### print(pd.unique(df['column_name'], return_counts=True)) ######### print(pd.isna(df['column_name']).sum()) ######### print(pd.notna(df['column_name']).sum()) ######### print(pd.fillna(df['column_name'], method='ffill')) ######### print(pd.dropna(df, how='any')) ######### print(pd.duplicated(df, keep=False)) ######### print(pd.drop_duplicates(df, inplace=True)) ######### print(pd.replace(df['column_name'], to_replace=['old_value1', 'old_value2'], value=['new_value1', 'new_value2'])) ######### print(pd.map(df['column_name'], lambda x: x * 2)) ######### print(pd.apply(df, lambda x: x.mean(), axis=1)) ######### print(pd.applymap(df, lambda x: str(x) + '_suffix')) ######### print(pd.select_dtypes(include=['object'])) ######### print(pd.select_dtypes(exclude=['object'])) ######### print(pd.set_option('display.float_format', '{:.2f}'.format)) ######### print(pd.reset_option('display.float_format')) ######### print(pd.style.use('default')) ######### print(pd.style.use('classic')) ######### print(pd.style.use('fivethirtyeight')) ######### print(pd.style.use('ggplot')) ######### print(pd.style.use('Solarize_Light2')) ######### print(pd.style.use('seaborn')) ######### print(pd.style.use('seaborn-bright')) ######### print(pd.style.use('seaborn-colorblind')) ######### print(pd.style.use('seaborn-dark')) ######### print(pd.style.use('seaborn-darkgrid')) ######### print(pd.style.use('seaborn-dark-palette')) ######### print(pd.style.use('seaborn-deep')) ######### print(pd.style.use('seaborn-muted')) ######### print(pd.style.use('seaborn-notebook')) ######### print(pd.style.use('seaborn-paper')) ######### print(pd.style.use('seaborn-pastel')) ######### print(pd.style.use('seaborn-poster')) ######### print(pd.style.use('seaborn-ticks')) ######### print(pd.style.use('seaborn-talk')) ######### print(pd.style.use('seaborn-white')) ######### print(pd.style.use('seaborn-whitegrid')) ######### print(pd.style.use('bmh')) ######### print(pd.style.use('fivethirtyeight')) ######### print(pd.style.use('grayscale')) ######### print(pd.style.use('dark_background')) ######### print(pd.style.use('dark_background')) ######### print(pd.style.use('fast')) ######### print(pd.style.use('fast')) ######### print(pd.read_csv(
- What long-term strategies are needed to address the teacher shortage and improve working conditions in North Rhine-Westphalia's schools?
- The long-term consequences of this teacher shortage could include larger class sizes, reduced educational quality, and potential burnout among remaining staff. The government's response focuses on ongoing efforts to alleviate teacher workload, but the survey results indicate these measures may be insufficient. Addressing systemic issues, like workload distribution and teacher support, is crucial for long-term teacher retention.
Cognitive Concepts
Framing Bias
The headline and introductory paragraph emphasize the high percentage of teachers considering leaving, framing the situation as a crisis. The article's structure prioritizes the union's concerns and statistics about workload, reinforcing a narrative of teacher burnout and dissatisfaction. The Minister's response is presented later and receives less emphasis.
Language Bias
The language used is largely neutral, although phrases like "den Job aufzugeben" (to give up the job) and "an den Nagel zu hängen" (to hang up the teacher's profession) might carry slightly negative connotations. However, these are relatively mild and common expressions.
Bias by Omission
The article focuses heavily on the teacher's perspective and the union's claims, but omits perspectives from school administration, students, or parents. The impact of teacher shortages on student learning is not directly addressed. While acknowledging the Minister's response, the article doesn't delve into the specifics of the implemented measures or their effectiveness. This omission limits the reader's ability to fully assess the situation.
False Dichotomy
The article presents a somewhat false dichotomy by highlighting the high percentage of teachers considering leaving, without sufficiently exploring the complexities of the issue. It doesn't fully examine other factors that may contribute to teacher satisfaction or dissatisfaction beyond workload.
Gender Bias
While the article uses gender-neutral language (e.g., Lehrerinnen und Lehrer), it doesn't delve into potential gendered aspects of the workload or whether there are differences in burnout rates between male and female teachers. This omission prevents a complete analysis of gender bias.
Sustainable Development Goals
The article highlights that over half of the teachers in North Rhine-Westphalia are considering leaving their jobs due to excessive workload and overtime. This directly impacts the quality of education as it leads to teacher shortages, increased stress among educators, and potentially compromises the quality of teaching and learning. The high number of teachers considering leaving the profession threatens the sustainability of the education system and the achievement of SDG 4 (Quality Education) targets related to ensuring inclusive and equitable quality education and promoting lifelong learning opportunities for all.