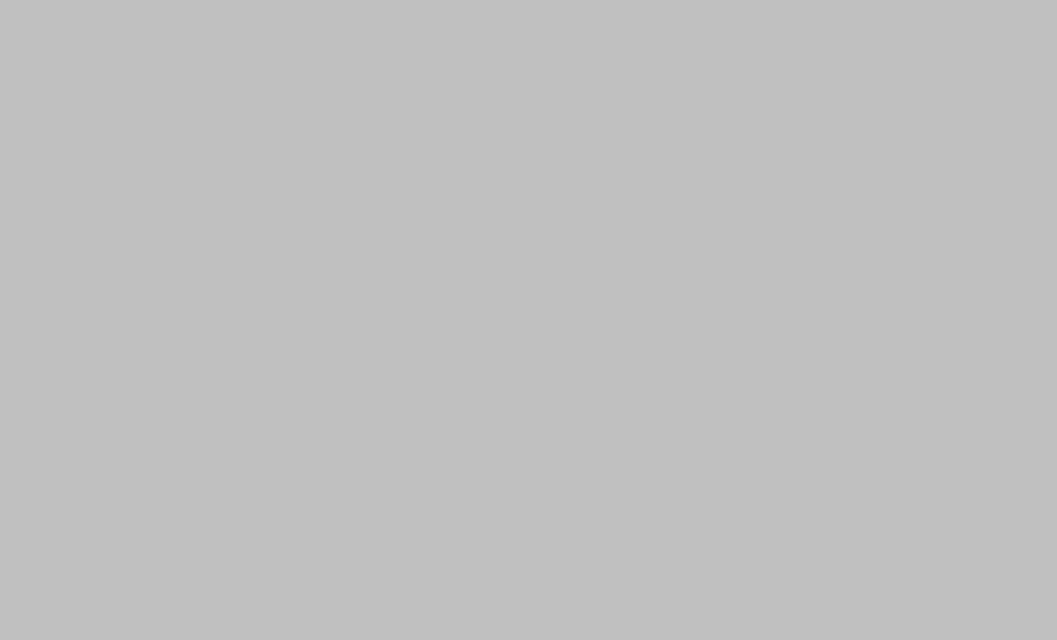
theguardian.com
UK Government AI Welfare Fraud System Shows Bias
The UK government's AI system for detecting welfare fraud shows bias against specific groups based on age, disability, marital status, and nationality, according to an internal assessment released under the Freedom of Information Act; although final decisions are made by humans, concerns remain about fairness and transparency.
- What specific demographic groups are disproportionately targeted by the UK government's AI-driven welfare fraud detection system, and what are the immediate consequences?
- The UK government's AI system for detecting welfare fraud shows bias based on age, disability, marital status, and nationality, as revealed by an internal assessment. This resulted in disproportionate targeting of certain groups for fraud investigations. The Department for Work and Pensions (DWP) admitted a \"statistically significant outcome disparity\" in a fairness analysis.
- What systemic changes are needed to ensure fairness and transparency in the government's use of AI for welfare fraud detection, and what are the potential long-term impacts of failing to address these issues?
- The government's 'hurt first, fix later' approach, evidenced by the rollout of biased AI despite lacking comprehensive fairness analysis, risks exacerbating existing inequalities. The lack of transparency regarding which specific groups are disproportionately targeted fuels public distrust and calls for greater accountability in government AI use. This situation highlights the urgent need for robust fairness assessments before deploying such systems.
- How does the DWP's assertion that human intervention mitigates AI bias reconcile with the acknowledged statistically significant disparities found in the fairness analysis, and what are the broader implications of this approach?
- This bias was discovered in a February 2024 fairness analysis of the universal credit advance system. Although the DWP claims human oversight mitigates risk, no analysis exists for bias related to race, sex, sexual orientation, religion, pregnancy, maternity, or gender reassignment. This lack of comprehensive analysis raises concerns about potential harm to marginalized groups.
Cognitive Concepts
Framing Bias
The article frames the AI bias as a significant issue, highlighting concerns about fairness and transparency. However, the DWP's perspective is also presented, suggesting the system is a necessary tool to combat fraud. This balanced framing avoids overly sensationalizing the issue while still emphasizing the importance of addressing the biases found.
Language Bias
The language used is largely neutral and objective, using terms like "statistically significant outcome disparity" and "fairness analysis". However, phrases like "hurt first, fix later" carry a charged connotation, suggesting criticism of the government's approach. While this phrase is attributed to a campaigner, the framing may influence the reader's perception.
Bias by Omission
The analysis fails to specify which age groups, disability statuses, or nationalities are disproportionately targeted by the algorithm. This omission prevents a full understanding of the AI's impact and hinders efforts to mitigate the harm. The redaction of this information, while offered to prevent fraud, arguably limits transparency and public accountability.
False Dichotomy
The article presents a false dichotomy by implying that either the AI system is completely unbiased or it causes immediate discrimination. The reality is more nuanced; the system shows bias in some areas while the final decision rests with a human, creating a complex situation that is not fully explored.
Gender Bias
The analysis does not assess gender bias. The lack of information on the algorithm's potential gendered effects is a significant omission, especially given the focus on other demographic biases.
Sustainable Development Goals
The AI system used to detect welfare fraud shows bias based on age, disability, marital status, and nationality, leading to unfair targeting of specific groups and potentially exacerbating existing inequalities. This contradicts the SDG target of reducing inequalities within and among countries.