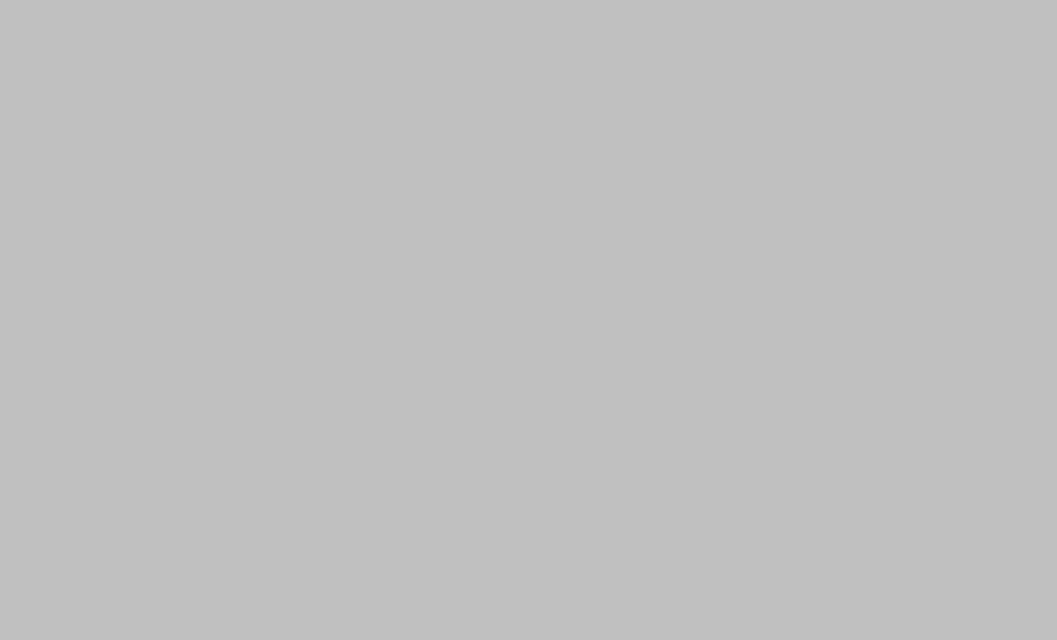
us.cnn.com
Workday Faces Lawsuit Alleging Age Discrimination in AI-Powered Hiring
A California judge's preliminary order allows a collective action lawsuit against Workday to proceed, alleging that its AI-powered applicant screening technology discriminates against applicants over 40, potentially setting a legal precedent for the use of AI in hiring.
- How does the Workday lawsuit exemplify broader concerns about AI bias in hiring processes?
- The lawsuit claims Workday's algorithm disproportionately rejects applicants over 40, highlighting concerns about AI bias in hiring. Plaintiffs cite rapid automated rejections, sometimes with inaccurate reasons, suggesting a lack of human review. The case underscores the potential for AI to perpetuate existing discrimination, even without explicit instructions.
- What are the immediate implications of the California judge's decision to allow the Workday lawsuit to proceed as a collective action?
- A California judge's preliminary order allows a collective action lawsuit against Workday to proceed, alleging its applicant screening technology discriminates against older applicants. Four plaintiffs over 40 claim algorithmic rejections from hundreds of applications, often within hours of submission. This ruling could set a precedent for AI use in hiring.
- What potential long-term impacts could this lawsuit have on the use of AI in recruitment and hiring practices across various industries?
- This case's outcome will significantly impact how companies utilize AI in hiring. A finding against Workday could trigger regulatory scrutiny and industry-wide changes in algorithmic hiring practices. Companies may need to implement stricter bias mitigation strategies and increase human oversight to avoid similar legal challenges.
Cognitive Concepts
Framing Bias
The article's headline and introduction emphasize the lawsuit and the allegations of discrimination, framing Workday as the defendant from the outset. While it presents Workday's denial, the framing leans towards portraying the company's technology as potentially discriminatory. The inclusion of examples of bias in other AI systems further strengthens this framing.
Language Bias
The language used is largely neutral, focusing on factual reporting of the lawsuit and expert opinions. However, terms like "disproportionately disqualifies" and "enormous danger" in reference to the AI technology carry a slightly negative connotation, subtly influencing reader perception.
Bias by Omission
The article focuses heavily on the lawsuit and the allegations, but doesn't explore in detail Workday's specific algorithms or the data used to train them. It mentions the potential for bias in AI hiring tools generally, but lacks specific examples of how Workday's algorithms might be biased beyond the plaintiffs' claims. This omission limits a complete understanding of how the algorithm functions and whether the biases are inherent in the design or a result of biased data.
False Dichotomy
The article presents a somewhat simplified view of the issue, focusing primarily on the dichotomy of AI-driven hiring being either entirely discriminatory or entirely meritocratic. It doesn't adequately address the complexities and nuances of AI in HR, such as the potential for mitigating bias through careful algorithm design and data selection.
Gender Bias
The article mentions gender bias in AI hiring tools generally, citing the Amazon example. However, the focus of the article is on age discrimination in the context of the Workday lawsuit. There is no specific analysis of gender bias within Workday's practices or the lawsuit itself.
Sustainable Development Goals
The lawsuit alleges that Workday's AI-powered hiring tools discriminate against older workers, exacerbating existing age inequality in the workplace. The algorithm's bias, even if unintentional, disproportionately affects individuals over 40, hindering their access to employment opportunities and perpetuating economic disparities. The case highlights the risk of AI systems reinforcing existing societal biases, thereby undermining efforts to reduce inequality.