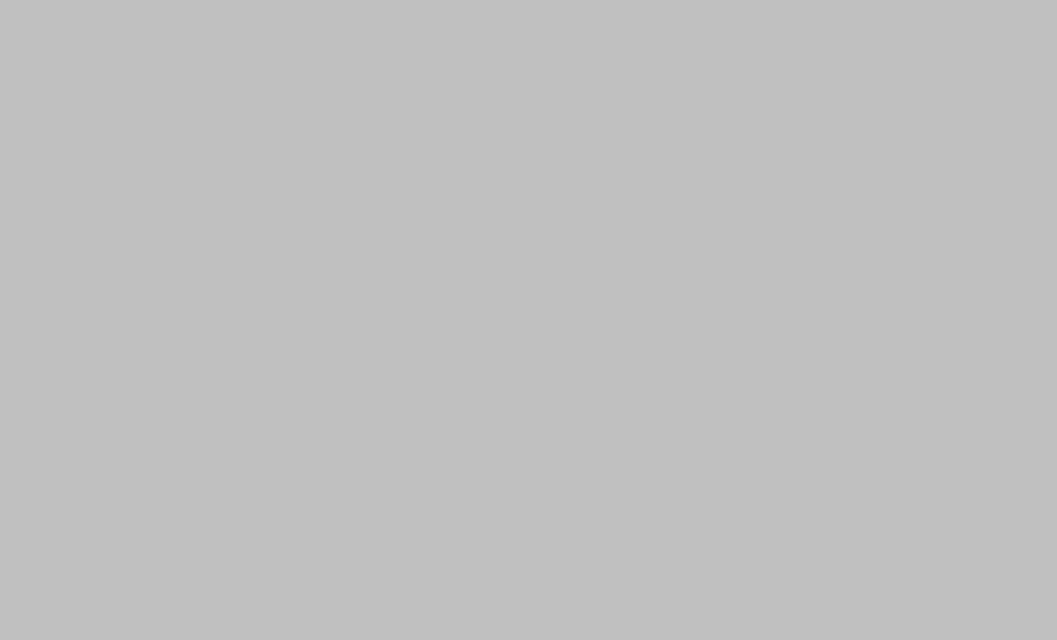
bbc.com
AI Blood Tests Show Promise in Early Ovarian Cancer Detection
New AI-powered blood tests are showing promise in detecting ovarian cancer five years before symptoms appear, improving diagnosis and survival rates; AI also speeds up pneumonia diagnosis and antibiotic selection, reducing costs and improving patient care.
- How are AI-powered blood tests revolutionizing early ovarian cancer detection and improving patient outcomes?
- AI-powered blood tests are emerging for early ovarian cancer detection, potentially reducing mortality by enabling diagnosis five years before symptoms appear. These tests analyze blood samples using nanotubes and AI to identify subtle molecular patterns indicative of cancer, surpassing the accuracy of existing biomarkers.
- What challenges hinder the development and widespread implementation of AI-driven diagnostic tools for rare cancers like ovarian cancer?
- The rarity of ovarian cancer and data siloing in hospitals hinder AI algorithm training. However, initial results show promising accuracy using limited data, suggesting significant improvement with increased data availability. This highlights the need for collaborative data sharing to optimize AI's diagnostic potential.
- What are the broader implications of AI-driven diagnostics for healthcare, considering both technological advancements and data-sharing challenges?
- AI's application extends beyond cancer, accelerating pneumonia diagnosis by identifying pathogens within 24 hours and guiding antibiotic selection. This reduces testing costs and improves patient outcomes, demonstrating AI's transformative impact across various medical fields. Future advancements may lead to AI-driven triage systems for gynecological diseases, providing faster and more accurate diagnoses.
Cognitive Concepts
Framing Bias
The article frames AI as a largely positive and revolutionary force in medical research, emphasizing its potential to improve cancer detection and speed up diagnostic processes. While acknowledging some challenges, the overall narrative strongly favors the optimistic perspective of AI's transformative capabilities. The headline (if any) and introduction would likely reinforce this positive framing.
Language Bias
The language used is generally neutral and objective, although terms like "rare, underfunded, and deadly" when describing ovarian cancer might be considered emotionally charged. The use of phrases such as "Hail Mary pass" to describe the use of limited data in algorithm training adds a subjective element. While aiming for accessible language, some neutral alternatives could be considered to ensure more objective tone.
Bias by Omission
The article focuses heavily on AI's role in early detection and faster diagnosis, but omits discussion on the potential limitations or drawbacks of AI-driven medical diagnostics, such as algorithmic bias, data privacy concerns, and the potential for misdiagnosis. While acknowledging data scarcity as a challenge, it doesn't delve into the ethical implications of using limited datasets to train algorithms. The high cost of some AI-driven tests is mentioned but not analyzed in detail in relation to healthcare access and affordability.
False Dichotomy
The article presents a somewhat simplistic view of AI's impact, contrasting its potential benefits with current limitations without fully exploring the nuances or complexities. For example, the challenges of data scarcity are mentioned, but the article doesn't discuss alternative approaches or strategies to mitigate this issue, such as data augmentation or federated learning.
Gender Bias
The article features several male and female experts, and there is no overt gender bias in the language used to describe them or their contributions. However, a deeper analysis might reveal implicit biases in the selection of quotes or the emphasis given to certain perspectives.
Sustainable Development Goals
The article highlights the development of AI-powered blood tests for early detection of ovarian cancer and faster diagnosis of pneumonia. Early detection significantly improves treatment outcomes and survival rates for ovarian cancer, while rapid pneumonia diagnosis ensures timely and effective antibiotic treatment, reducing mortality and healthcare costs. These advancements directly contribute to improved health and well-being.