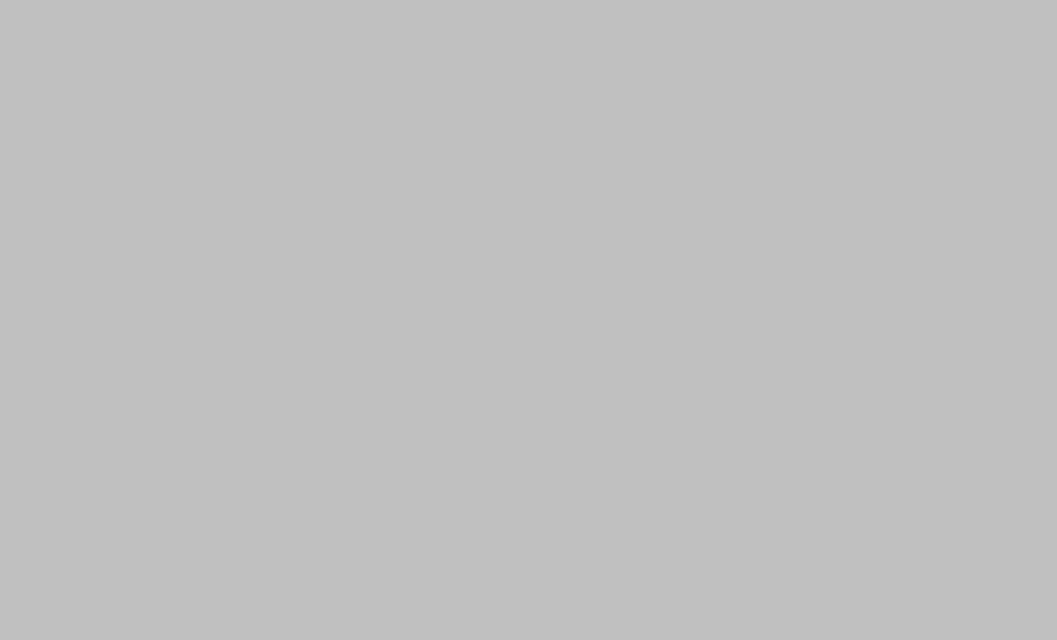
africa.chinadaily.com.cn
China's AI Talent Gap: Challenges and Reforms
China's AI education system faces a significant talent shortage, with outdated curriculums, limited industry experience among faculty, and insufficient university-industry collaboration hindering its national AI strategy; reforms are needed to bridge this gap and ensure future competitiveness.
- What are the key challenges facing China's AI education system, and how significantly do these impact its national AI strategy and economic competitiveness?
- China faces a critical shortage of AI professionals, with estimates suggesting a shortfall of up to 4 million by 2030. This lack of skilled workers hinders national AI strategies and economic growth. Current AI education struggles to keep pace with technological advancements, resulting in graduates lacking practical skills.
- How does the current state of China's university-industry collaboration in AI compare to that of other countries (e.g., the US), and what are the main differences in approach and effectiveness?
- The gap between AI education and industry needs stems from several factors: outdated curriculums, insufficient industry experience among faculty, and limited university-industry collaboration. These issues are hindering the development of a skilled workforce needed to compete globally. China's AI education system needs substantial reform to bridge this divide.
- What specific policy changes and reforms are needed to improve the quality and relevance of AI education in China, and what are the potential long-term economic and geopolitical consequences of addressing these challenges?
- To cultivate a robust AI talent pipeline, China should implement a tiered training system, strengthen cross-disciplinary coursework, and foster stronger industry-academia partnerships. This includes creating industry-academia innovation labs, providing faculty with industry experience, and streamlining the process for turning research into commercial applications. These measures are crucial for future competitiveness.
Cognitive Concepts
Framing Bias
The framing is predominantly problem-oriented, focusing on the shortcomings of China's current AI education system. While solutions are offered, the overall tone emphasizes the urgency and severity of the challenges, potentially creating a sense of crisis or alarm. The headline (if any) would heavily influence this perception. The use of statistics, such as the projected shortfall of AI professionals, further emphasizes the negative aspects.
Language Bias
The language used is largely neutral and objective, relying on factual statements and statistical data. However, phrases like "critical to the success" and "worse" could be considered slightly loaded, but the overall tone remains informative and avoids overly emotional or subjective language.
Bias by Omission
The analysis focuses primarily on the challenges and solutions within China's AI education system. While it mentions the US system as a point of contrast, a more in-depth comparison of different national approaches to AI education and talent development would provide a richer context. Additionally, the perspectives of AI professionals themselves, students, and industry leaders are absent, limiting a full understanding of the issue. The omission of global trends beyond China and the US also limits the scope of the analysis.
False Dichotomy
The article presents a somewhat simplified view of the relationship between universities and industry. While it highlights the need for stronger collaboration, it doesn't fully explore the complexities and potential challenges in forging effective partnerships between these two sectors. The solutions proposed assume a relatively straightforward implementation, overlooking potential obstacles like bureaucratic hurdles or conflicting priorities.
Sustainable Development Goals
The article highlights the need for improved AI education to meet industry demands and global competition. Improving AI curriculums, faculty training, and industry-academia collaboration directly contributes to SDG 4 (Quality Education) by ensuring a skilled workforce equipped for the future job market. The proposed solutions, such as a tiered talent training system, cross-disciplinary coursework, and industry-academia innovation labs, are all aimed at enhancing the quality of AI education.