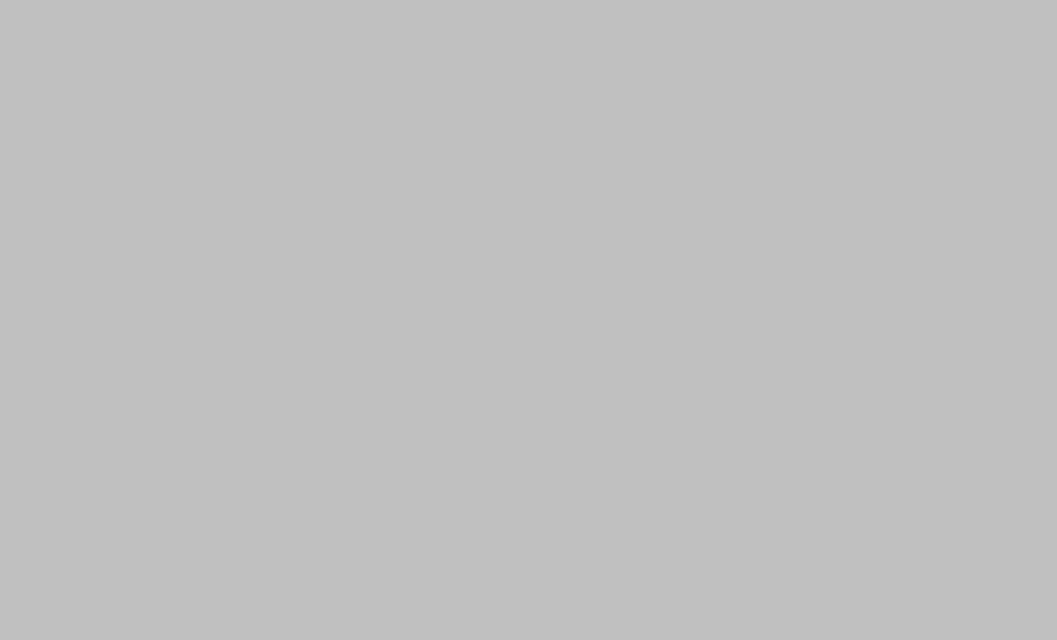
nrc.nl
GPT-NL: A Dutch AI Model Faces Delays Due to Data Scarcity
The Dutch AI model GPT-NL, developed by TNO, NFI, and SURF, is facing delays due to data scarcity, with its training delayed until June 2023 instead of summer 2022, aiming to provide a privacy-focused alternative to large language models developed by American and Chinese tech giants.
- How does GPT-NL's approach to data acquisition and usage differ from major competitors, and what are the trade-offs?
- The project's delay highlights the difficulty of building a large language model ethically and with limited resources. While GPT-NL prioritizes data privacy by using only consented, anonymized Dutch data, this approach significantly restricts the data volume compared to competitors like Meta's Llama, impacting model performance and training time.
- What are the primary challenges facing GPT-NL's development, and how do these affect its timeline and potential impact?
- GPT-NL, a Dutch AI model developed by TNO, NFI, and SURF, aims to provide a privacy-respecting alternative to models like ChatGPT. Its development, however, is facing delays due to data acquisition challenges; the training phase, initially slated for summer 2022, is now set to begin in June 2023.
- What are the broader implications of GPT-NL's success or failure for European technological sovereignty and the ethical development of AI?
- The success of GPT-NL hinges on attracting more data contributors by the April deadline. The project's current funding of €12.5 million over five years, along with its commitment to ethical data handling, presents both a challenge and a unique selling proposition in the global AI landscape. Future success will depend on navigating this data scarcity while maintaining its ethical standards.
Cognitive Concepts
Framing Bias
The narrative frames GPT-NL as an underdog battling against tech giants, emphasizing its challenges and resource constraints. The headline (if there was one) and introduction likely highlight the delays and difficulties, potentially setting a negative expectation. This framing, while relatable, might overshadow the project's long-term goals and potential significance.
Language Bias
The language used is generally neutral, but phrases like "the project runs smoothly," "difficult," and "frustrating" could be considered slightly loaded, conveying a sense of struggle. More neutral alternatives could include "the project faces challenges," "complex," and "demanding." The description of GPT-NL as "David against Goliath" is a clear metaphor that frames the project in a sympathetic light.
Bias by Omission
The article focuses heavily on the challenges faced by GPT-NL, such as data scarcity and delays, potentially downplaying the project's ambition and potential benefits. While it mentions the ethical considerations, it omits a balanced perspective on the trade-offs between ethical data sourcing and the scale of other AI models. The article also lacks details on the specific types of data already collected and how the data cleaning process works. This omission could influence the reader's understanding of the project's progress and feasibility.
False Dichotomy
The article presents a somewhat false dichotomy between GPT-NL's ethical approach and the large-scale, less ethical approaches of other AI models. It implies that one must choose between ethical data sourcing and achieving comparable performance, overlooking the possibility of finding a middle ground or alternative strategies.
Sustainable Development Goals
The development of GPT-NL, a Dutch AI model, aims to provide a responsible and ethical alternative to existing AI models. This aligns with Quality Education by promoting access to information and fostering innovation in the field of AI, while emphasizing ethical considerations in its development and use. The project also indirectly supports education through the potential use of the model in educational settings.